AI-enabled medical devices: Is current usability practice fit for purpose?
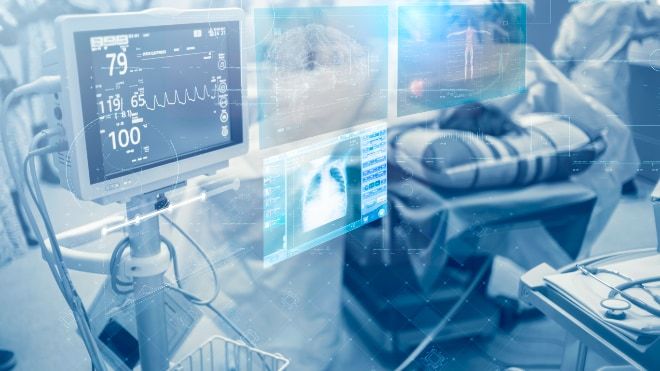
This article provides three observations on how usability/human factors engineering could be more effectively applied to the design, development and regulation of AI-enabled medical devices.
The Background
Software incorporating artificial intelligence (AI), including the subset of AI known as machine learning (ML), is increasingly being used in medical devices. It has been reported that AI has the potential to transform healthcare by deriving new and important insights from the vast amount of data generated during the delivery of healthcare every day [1].
Example applications of AI include earlier disease detection, more accurate diagnoses, and mental health chatbots [e.g. 2-4]. Recent FDA-approved AI medical devices include a device that assists in the detection of heart failure [5] and a device that analyses health data to predict serious outcomes from COPD [6].
Given the highly iterative, autonomous and adaptive nature of those technologies, traditional paradigms on medical device design, development and regulation are being refined and updated. Over the last few years, regulatory bodies have published action plans and various guidance documents to address the unique challenges posed by AI [1, 7-11].
One key challenge is the extent to which AI will effectively integrate into existing clinical systems, which comprise of a myriad of complex interactions between people and technology – known as the ‘sociotechnical system’ [12]. This body of work is addressed by usability/human factors engineering (UE/HFE) [13-18] – a discipline which is arguably the key enabler for unlocking the potential of AI.
Many of the issues to be addressed by the UE/HFE community are now well documented [19, 20]. Issues include (i) the impact of AI on users’ workload and situational awareness, (ii) the risk of automation bias (relying uncritically on the AI), (iii) the need for transparency and affordance to ensure users understand the performance, behaviour and potential bias of AI medical devices, and (iv) the delivery of training (and retraining when AI medical devices adapt) to ensure users have the appropriate knowledge and skills to use AI safely.
The question posed by this article is whether existing UE/HFE methods, including the documentation prepared by manufactures for notified and regulatory bodies, are adequate to ensure AI devices are safe and effective to use, and integrate well into the clinical system.
Observation 1: The context of use needs expanded
To comply with IEC 62366 [13,14], manufacturers need to prepare a ‘use specification’ which provides details on the ‘context of use’. Characteristics of intended users and use environments, as well as other details, need to be defined. The use specification, which provides an input into all usability activities, is defined early during user research and updated iteratively as more insights are gathered from usability analysis and evaluations.
One area the use specification could expand upon is an improved characterisation of the existing clinical/sociotechnical system (the current standard of care) and an assessment of how this clinical system will change once the AI technology is deployed. For instance, automating clinical tasks that were previously manual has the potential to considerably impact on existing clinical workflows, teamworking, skills and education, and use of other devices/technology [21].
An autonomous infusion pump, for example, would replace the practice of double-checking by a second nurse; a practice which often serves as an opportunity for teaching and discussion. What impact would automation have on the patient’s wellbeing if contact between staff and the patient reduces? Would staffs’ workload decrease due to tasks being automated or increase due to monitoring and administration of configurable elements?
We propose expanding the use specification to include more details on the changes AI will have on the clinical system. Forming part of an impact assessment, one solution would be to conduct a simplified ‘comparative task analysis’ comparing the workflow of the current standard of care with the envisaged workflow once the new medical device is deployed in the clinician system. Differences between those workflows could be clearly visualised, providing a foundation on which to analyse and evaluate sociotechnical integration issues in more detail.
Observation 2: ‘Knowledge tasks’ require greater attention
In the FDA’s primary guidance on HFE [15], the term ‘knowledge task’ was introduced to refer to critical tasks related to users’ knowledge – tasks that cannot be evaluated simply by observing users interact with the device. Examples of knowledge tasks include users’ understanding of (i) warnings and/or contraindications, (ii) components that are disposable and not for reuse, (iii) the risks of taking shortcuts, and (iv) the need to perform periodical cleaning and maintenance of the device.
AI-enabled devices will arguably require greater attention to knowledge tasks compared with other types of medical devices. For instance, users will need to understand (i) the inputs and the outputs of the algorithm, (ii) the degree of automation being used, (iii) what elements of the device are configurable, (iv) the performance and limitations of the algorithm, (v) the model architecture, (vi) the changes made to the algorithm, and (vii) any metrics or visualisations being presented.
We feel that more clarity is needed in the literature on how best to analyse and evaluate knowledge tasks, especially for AI medical devices. For instance, how should knowledge tasks be represented in task analysis, and what unique questions should be asked in usability studies to ensure all aspects of AI functions are understood?
In task analysis activities, we recommend that the knowledge needed to perform ‘critical tasks’ should be more explicitly stated – often the emphasis is only on describing the tasks users perform. Further, in usability evaluations/studies, we feel an industry-recognised AI topic guide or toolkit could assist practitioners with evaluating knowledge tasks (e.g. questions to evaluate the risk of automation bias).
Lastly, as a side (and perhaps pedantic) issue, we don’t feel the industry-adopted term ‘knowledge task’ is particularly helpful given that users require knowledge to perform all tasks, including those which are observable. ‘Task knowledge’ is perhaps more suitable to encourage manufacturers to consider the knowledge needed to perform any task, with an emphasis on those which are critical to safety.
Observation 3: Refining and making better use of well-established UE/HFE tools
As stipulated earlier in this article, AI-enabled medical devices pose a range of UE/HFE challenges – for instance, the impact of AI on users’ workload and situational awareness. Many of those issues are not new. They are well documented in other industries and a wide range of human factors tools and techniques exist to evaluate them.
Examples of situational awareness assessment techniques include the SA Rating Technique (SART) [22] and SA Global Assessment Technique (SAGAT) [23], and a well-known workload assessment technique is NASA TLX [24]. However, in the medical device industry, many of those tools and techniques are underutilised and/or very little reference is made to them in the literature.
There is also scope for tailoring techniques already in use in the medical device industry. For instance, a unique set of heuristics (or best practice principles) could be developed for conducting a heuristic evaluation of an AI medical device. Similarly, a unique set of AI-based questions could be developed for conducting a cognitive walkthrough.
Lastly, one area which the UE/HFE community needs to better understand is the concept of ‘human-AI teaming’ – the 7th guiding principle of the Good Machine Learning Practice for Medical Device Development [9]. As AI becomes more advanced and powerful – having the capacity to learn, make decisions and adapt – we need to think of AI as the new team member rather than seeing AI in isolation.
To be an effective team member, AI needs to exhibit desirable behaviours that are constructive to teamwork. In one model of teamwork, key teamwork behaviours consist of leadership, mutual performance monitoring, back-up behaviour, adaptability and team orientation [25]. Questions for the UE/HFE community are: (i) how should teamwork performance be evaluated in usability studies? (ii) can AI medical devices commit use errors and, if so, how should they be captured? and (iii) should acceptance criteria for summative evaluations be reflective of the team’s performance rather than on the individual’s performance?
Conclusions
This article has highlighted areas where UE/HFE could be more effectively applied to the design, development and regulation of AI-enabled medical devices – however, many of the observations in this article are applicable to all types of devices.
As AI technologies become more powerful and advanced, it is important that UE/HFE tools – and the way in which activities are documented – remain up to date and relevant so that the full potential of AI is realised.
References
[1] FDA (2024) Marketing Submission Recommendations for a Predetermined Change Control Plan for Artificial Intelligence-Enabled Device Software Functions. Guidance for Industry and Food and Drug Administration Staff. December 4, 2024.
[2] Beede E, Baylor E, Hersch F, et al., 2020. A Human-Centered Evaluation of a Deep Learning System Deployed in Clinics for the Detection of Diabetic Retinopathy. Proceedings of the 2020 CHI Conference on Human Factors in Computing Systems: Association for Computing Machinery; 1–12.
[3] Zeltzer D, Herzog L, Pickman Y, Steuerman Y, Ilan Ber R, Kugler Z, Shaul R, Ebbert JO (2023) Diagnostic Accuracy of Artificial Intelligence in Virtual Primary Care, Mayo Clinic Proceedings: Digital Health, Volume 1, Issue 4, 2023, Pages 480-489.
[4] Rollwage M, Juchems K, Pisupati S, Prichard G, Balogh A, McFadyen J, et al. The Limbic Layer: transforming large language models (llms) into clinical mental health experts. PsyArXiv. Preprint posted online November 27, 2024.
[5] Ultromics (2022) Ultromics receives FDA clearance for its breakthrough device for HFpEF detection. https://www.ultromics.com/press-releases/ultromics-receives-fda-clearance-for-its-breakthrough-device-echogo-heart-failure, 6 Dec 2022.
[6] Lenus Stratify – an early detection of clinical risks at a patient and population level across a range of chronic conditions including COPD and Heart Failure. https://lenushealth.com/solutions/lenus-stratify.
[7] FDA (2025) Artificial Intelligence-Enabled Device Software Functions: Lifecycle Management and Marketing Submission Recommendations. Draft Guidance for Industry and Food and Drug Administration Staff. January 7, 2025.
[8] FDA (2021) Artificial Intelligence/Machine Learning (AI/ML)-Based Software as a Medical Device (SaMD) Action Plan. January 2021.
[9] FDA, Health Canada & MHRA (2021) Good Machine Learning Practice for Medical Device Development: Guiding Principles. October 2021.
[10] BSI & AAMI (2020). Machine Learning AI in Medical Devices. Adapting Regulatory Frameworks and Standards to Ensure Safety and Performance.
[11] BSI, MHRA & AAMI (2019) The emergence of artificial intelligence and machine learning algorithms in healthcare: Recommendations to support governance and regulation. Position paper.
[12] Carayon, P. (2013). Sociotechnical systems approach to healthcare quality and patient safety. Work. 2012;41(0 1):3850–3854.
[13] IEC 62366-1:2015+AMD1:2020 Medical devices – Part 1: Application of usability engineering to medical devices.
[14] IEC 62366-2:2016 Medical devices – Part 2: Guidance on the application of usability engineering to medical devices. Edition 1.0.
[15] FDA (2016) Applying Human Factors and Usability Engineering to Medical Devices. Guidance for Industry and Food and Drug Administration Staff. February 3, 2016.
[16] FDA (2022) Content of Human Factors Information in Medical Device Marketing Submissions. Draft Guidance for Industry and Food and Drug Administration Staff. December 9, 2022.
[17] MHRA (2021) Human Factors and Usability Engineering – Guidance for Medical Devices Including Drug-device Combination Products, Version 2.0, January 2021.
[18] ANSI/AAMI HE75: 2009/(R)2018. Human Factors Engineering – Design of Medical Devices. Annex A: Statistical Justification for Sample Sizes in Usability Testing.
[19] Sujan, M., Pool, R. Salmon, P. (2022) Eight human factors and ergonomics principles for healthcare artificial intelligence. BMJ Health & Care Informatics. Volume 29, Issue 1.
[20] Choudhury, A, Asan, O. (2022) Impact of accountability, training, and human factors on the use of artificial intelligence in healthcare: Exploring the perceptions of healthcare practitioners in the US. Human Factors in Healthcare 2.
[21] Bainbridge, L. (1983) Ironies of Automation. Automatica, 19(6), pp. 775-779.
[22] F. T. Durso, and S. D. Gronlund, ‘Situation awareness’, Handbook of Applied Cognition, 1999, pp.283-314.
[23] M. R. Endsley, “Situation awareness global assessment technique (sagat),” in Aerospace and Electronics Conference, NAECON 1988, May 1988, Proceedings of the IEEE 1988 National, pp. 789-795.
[24] Hart SG, Staveland LE (1988). Development of NASA-TLX (Task Load Index): Results of Empirical and Theoretical Research. In Hancock, Peter A.; Meshkati, Najmedin (eds.). Human Mental Workload. Advances in Psychology. Vol. 52. Amsterdam: North Holland. pp. 139–183.
[25] Salas E, Sims DE, Burke CS. (2005) Is there a “Big Five” in Teamwork? Small Group Research. October 2005; 36:555 -599.